- Published on
Dolphin-2.2.1-mistral-7b Fullstack Application Guide
- Authors
- Name
- Tim Dolan
Crafting an AI-Powered Chatbot with dolphin-2.2.1-mistral-7b
In today's post, we're going to dive into how we can harness the capabilities of FastAPI and Hugging Face's Transformers library to build an interactive chatbot. FastAPI is a modern, fast (high-performance) web framework for building APIs with Python 3.7+ based on standard Python type hints.
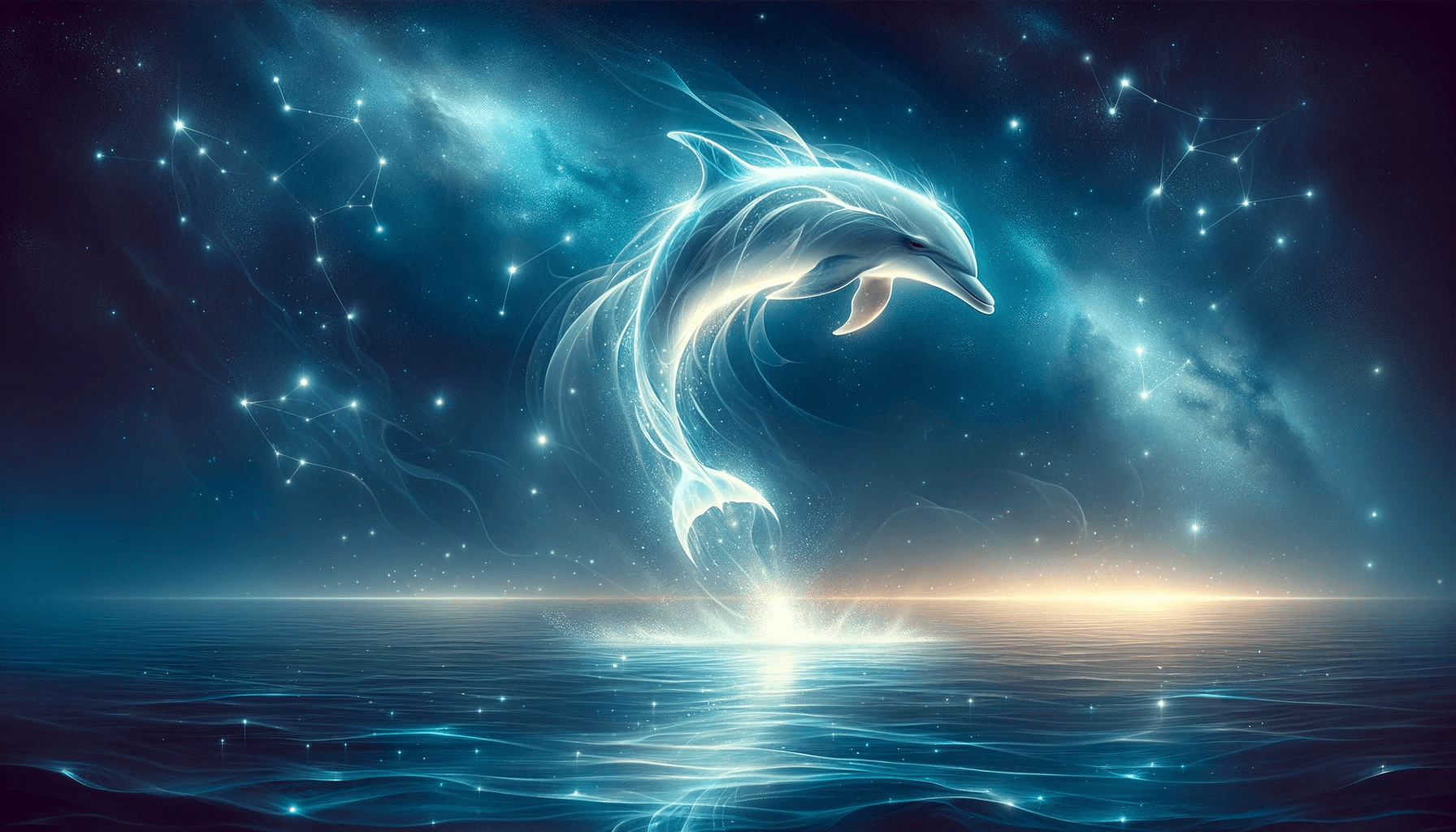
Import the Libraries
The first thing you will need to do is make sure you have the required libraries installed.
- Transformers 4.35.0.dev0
pip install transformers --upgrade
If this command doesn't work you can try to install from the source code.
pip install git+https://github.com/huggingface/transformers
- Pytorch 2.0.1+cu118
conda install pytorch torchvision torchaudio pytorch-cuda=12.1 -c pytorch-nightly -c nvidia
The remainder of the requirements are:
pip install fastapi uvicorn streamlit pydantic tokenizers datasets einops sentencepiece
from fastapi import FastAPI, HTTPException, WebSocket, Request
from transformers import pipeline
from fastapi.middleware.cors import CORSMiddleware
from pydantic import BaseModel, Field
from typing import Optional, List
import torch
import re
Initializing the FastAPI Application
Our journey begins with the creation of an instance of FastAPI:
app = FastAPI()
This instance is our application, which will listen for incoming web requests and route them to the appropriate handler functions.
Preparing the AI Model
Before we can handle any requests, we need to set up our AI model, which will generate the chatbot's responses:
device = torch.device("cuda" if torch.cuda.is_available() else "cpu")
pipe = pipeline(
"text-generation",
model="ehartford/dolphin-2.2.1-mistral-7b",
torch_dtype=torch.bfloat16,
device_map=device,
)
Here, we're configuring the device to use GPU acceleration with CUDA if available; otherwise, we default to CPU. The pipeline is initialized with a specific text-generation model that will run on the designated device.
Configuring Cross-Origin Resource Sharing (CORS)
To ensure that our API can be accessed from web pages served by different origins, we configure CORS:
origins = [
"http://localhost",
"http://localhost:8000",
"http://localhost:8501",
# Additional origins can be added here
]
app.add_middleware(
CORSMiddleware,
allow_origins=origins,
allow_credentials=True,
allow_methods=["*"],
allow_headers=["*"],
)
This CORS configuration is crucial when our API is consumed by clients from various domains, providing both flexibility and control over who can access our API.
Defining the Data Model
We use Pydantic models to define the structure of requests our API can handle:
class QueryModel(BaseModel):
user_message: str
max_new_tokens: int = 256
do_sample: bool = True
temperature: float = 0.7
top_k: int = 50
top_p: float = 0.95
system_message: Optional[str] = "You are a friendly chatbot that will answer any question."
This model describes the expected request body: a user message, various parameters to control text generation, and an optional system message.
Our endpoint is where the magic happens. We define a POST method that takes in the QueryModel:
@app.post("/dolphin/", description="Get a response from the dolphin chatbot with a custom system message. The default message is geared towards python code.")
async def get_custom_response(query: QueryModel):
# ... (processing code will go here)
The /dolphin/ endpoint is described and awaits incoming POST requests with data conforming to our QueryModel.
Handling the Chatbot Interation
Within get_custom_response, we process the data to generate a chatbot response:
messages = [
{"role": "system", "content": query.system_message},
{"role": "user", "content": query.user_message},
]
prompt = pipe.tokenizer.apply_chat_template(
messages, tokenize=False, add_generation_prompt=True
)
outputs = pipe(
prompt,
max_new_tokens=query.max_new_tokens,
do_sample=query.do_sample,
temperature=query.temperature,
top_k=query.top_k,
top_p=query.top_p
)
We construct a dialogue with system and user messages, then feed this into our text-generation pipeline.
Extracting and Cleaning the Response
After generating the text, we need to extract and clean it up before sending it back to the user:
try:
response = outputs[0]["generated_text"].split('[INST]')[-1].split('[/INST]')[0].strip()
except IndexError:
raise HTTPException(status_code=400, detail="Invalid response structure from model")
response = re.sub(r'<<SYS>>.*?<</SYS>>', '', response, flags=re.DOTALL)
response = re.sub(r'\|\|.*?\|\|', '', response).strip()
response = response.strip()
This code handles potential errors in the response structure, removes system messages, and strips out any unwanted formatting.
Sending the Response
Finally, we return the processed response as JSON:
return {"response": response}
The user receives a clean, understandable response from our chatbot.
Creating a Streamlit User Interface for Our Chatbot
Streamlit allows us to quickly create web apps for our machine learning projects with minimal code. We'll design a simple chat interface where users can send messages to the chatbot and view its responses.
Firstly, we set the page title and icon:
BASE_URL = "http://localhost:8000"
st.set_page_config(
page_title="Dolphin 2.2.1 (Uncensored)",
page_icon=":dolphin:", # You can specify a URL or an emoji as the tab icon
)
Adding a Title and Sidebar Configurations
We add a title to our app and configure the sidebar that will hold our parameters:
st.title("Dolphin 2.2.1 (Uncensored)")
# Sidebar configurations
st.sidebar.image("assets/thumbnail.png") ## Add a thumbnail image at this path or delete this line
st.sidebar.title("Parameters")
st.sidebar.info("Configure the model parameters and endpoint from the sidebar and send your message!")
Allowing Endpoint Selection
The user can select the desired endpoint from the sidebar. Currently, we only have one endpoint, "dolphin":
endpoints = ["dolphin"]
selected_endpoint = st.sidebar.selectbox("Choose Endpoint:", endpoints)
Customizing Model Parameters
Streamlit's sidebar allows users to customize the chatbot's behavior:
if selected_endpoint == "dolphin":
system_message = st.sidebar.text_input("System Message:", value="You are a friendly chatbot that will answer any question.")
max_new_tokens = st.sidebar.slider("Max New Tokens:", 50, 500, 256)
do_sample = st.sidebar.checkbox("Do Sample:", value=True)
temperature = st.sidebar.slider("Temperature:", 0.1, 1.0, 0.7, 0.1)
top_k = st.sidebar.slider("Top K:", 1, 100, 50)
top_p = st.sidebar.slider("Top P:", 0.1, 1.0, 0.95, 0.05)
These sliders and checkboxes allow users to adjust the generation settings for the model.
Managing Chat History
Streamlit can maintain a chat history within the session state:
if "messages" not in st.session_state:
st.session_state.messages = []
for message in st.session_state.messages:
with st.chat_message(message["role"]):
st.markdown(message["content"])
This code snippet ensures that messages persist across reruns of the app.
Handling User Input
We provide a chat input where the user can type their messages:
if prompt := st.chat_input("What is up?"):
# Sending the message to the backend and processing will go here.
When the user sends a message, it's processed and sent to the backend API.
Communicating with the Backend API
The user's input is sent to the API endpoint, and the response is handled as follows:
data = {
"user_message": prompt,
"max_new_tokens": max_new_tokens,
"do_sample": do_sample,
"temperature": temperature,
"top_k": top_k,
"top_p": top_p,
"system_message": system_message
}
url = f"{BASE_URL}/{selected_endpoint}"
response = requests.post(url, json=data)
response_data = response.json()
We craft a JSON payload from the user's input and model parameters, send a POST request to our FastAPI backend, and then receive the chatbot's response.
Displaying the Chatbot's Response
Lastly, the chatbot's response is displayed in a way that simulates typing:
with st.chat_message("user"):
st.markdown(prompt)
with st.chat_message("assistant"):
message_placeholder = st.empty()
full_response = ""
assistant_response = response_data["response"]
for chunk in assistant_response.split():
full_response += chunk + " "
time.sleep(0.05)
message_placeholder.markdown(full_response + "▌")
message_placeholder.markdown(full_response)
Running the App
Since you will need to run two servers with live updates simultaneously, you will also need to run a shell or a batch command to run them both at the same time.
#!/bin/bash
# Execute the streamlit command
streamlit run app.py &
# Execute the uvicorn command
uvicorn api:app --reload
Add this code in a file called run.sh
and run it in your terminal with bash run.sh
or ./run.sh
if you have the correct permissions.
If you have issues running the file, run this command first:
chmod +x run.sh
This will make the file executable.
Conclusion
The chatbot's message appears one word at a time, adding to the user's experience of talking to a live chatbot.
With Streamlit, we've now created a UI where users can interact with our AI-powered chatbot in a more natural and engaging way. This combination of a powerful backend API with an intuitive frontend makes our chatbot not only smart but also accessible to anyone with a web browser.
Stay tuned for more posts where we might explore additional features such as voice input, more advanced response handling, or integration with messaging platforms!